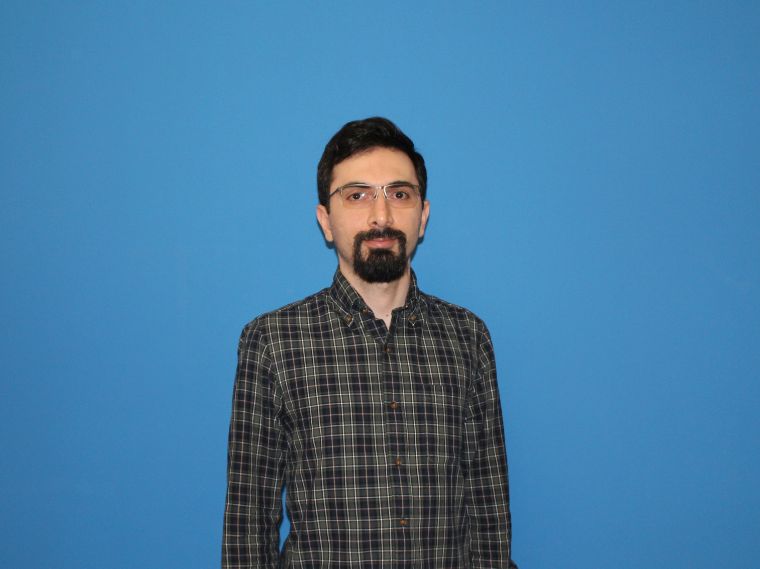
T
+34 946 567 842
F
+34 946 567 842
E
mabedi@bcamath.org
Information of interest
- Orcid: 0000-0001-6937-0243
Mohammad Mahdi Abedi is a postdoctoral researcher working with the simulation of wave propagation group at BCAM, where he is responsible for the development of deep learning and semi-analytical methods with seismic applications. He has a Ph.D. in Geophysics from University of Tehran, an M.Sc. in Petroleum Engineering, and a B.Sc. in Mining Engineering from the Amirkabir University of Technology.
-
Ensemble Deep Learning for Enhanced Seismic Data Reconstruction
(2024-05)Seismic data often contain gaps due to various obstacles in the investigated area and recording instrument failures. Deep-learning techniques offer promising solutions for reconstructing missing data parts by utilizing ...
-
Semi-blind-trace algorithm for self-supervised attenuation of trace-wise coherent noise
(2024-03-01)Trace-wise noise is a type of noise often seen in seismic data, which is characterized by vertical coherency and horizontal incoherency. Using self-supervised deep learning to attenuate this type of noise, the conventional ...
-
APPLICATION OF SPARSE DICTIONARY LEARNING TO SEISMIC DATA RECONSTRUCTION
(2023-04)According to the principle of compressed sensing (CS), under-sampled seismic data can be interpolated when the data becomes sparse in a transform domain. To sparsify the data, dictionary learning presents a data-driven ...
-
A Multidirectional Deep Neural Network for Self-Supervised Reconstruction of Seismic Data
(2022-12-06)Seismic studies exhibit gaps in the recorded data due to surface obstacles. To fill in the gaps with self-supervised deep learning, the network learns to predict different events from the recorded parts of data and then ...
-
THE EFFECT OF SUPERVISED FEATURE EXTRACTION TECHNIQUES ON THE FACIES CLASSIFICATION USING MACHINE LEARNING
(2022-12)The widely accepted supervised machine learning classification algorithms are used for the semi-automating of the feature extraction process. In the machine learning facies classification process, each wireline log is a ...
-
Nonhyperbolic normal moveout stretch correction with deep learning automation
(2022-02-15)Normal-moveout (NMO) correction is a fundamental step in seismic data processing. It consists of mapping seismic data from recorded traveltimes to corresponding zero-offset times. This process produces wavelet stretching ...
-
Approximations for traveltime, slope, curvature, and geometrical spreading of elastic waves in layered transversely isotropic media
(2021-10)Each seismic body wave, including quasi compressional, shear, and converted wave modes, carries useful subsurface information. For processing, imaging, amplitude analysis, and forward modeling of each wave mode, we need ...
-
Large-offset P-wave traveltime in layered transversely isotropic media
(2021-05-01)Large-offset seismic data processing, imaging, and velocity estimation require an accurate traveltime approximation over a wide range of offsets. In layered transversely isotropic media with a vertical symmetry axis, the ...
-
A MULTIDIRECTIONAL DEEP NEURAL NETWORK FOR SELF-SUPERVISED RECONSTRUCTION OF SEISMIC DATA
(2021)Seismic studies exhibit gaps in the recorded data due to surface obstacles. To fill in the gaps with self-supervised deep learning, the network learns to predict different events from the recorded parts of data and then ...
-
A new acoustic assumption for orthorhombic media
(2020-11)In exploration seismology, the acquisition, processing and inversion of P-wave data is a routine. However, in orthorhombic anisotropic media, the governing equations that describe the P-wave propagation are coupled with ...
-
Rational approximation of P-wave kinematics — Part 2: Orhorhombic media
(2020-09)Orthorhombic anisotropy is a modern standard for 3D seismic studies in complex geologic settings. Several seismic data processing methods and wave propagation modeling algorithms in orthorhombic media rely on phase-velocity, ...
-
Rational approximation of P-wave kinematics — Part 1: Transversely isotropic media
(2020-09)In seismic data processing and several wave propagation modeling algorithms, the phase velocity, group velocity, and traveltime equations are essential. To have these equations in explicit form, or to reduce algebraic ...