CAS
Computational and Applied Statistics
The aim of the research line in Applied Statistics is to create innovative statistical models, inference methods, computational algorithms and visualization tools for analyzing complex data sets from different and diverse sources.
The aim of the research in Applied Statistics is to consolidate BCAM as a reference in areas such as biostatistics, demography, environmental modeling, medical statistics, epidemiology, business analytics, and biomedical research applications involving data-driven mathematical and statistical tools. We aim to capture opportunities and challenges empowering collaboration with other research areas and groups (other BERC centers, business collaborators, Public Health institutions, government organizations, and Universities) in accessing, managing, integrating, analyzing and modeling datasets of diverse nature and complexity.
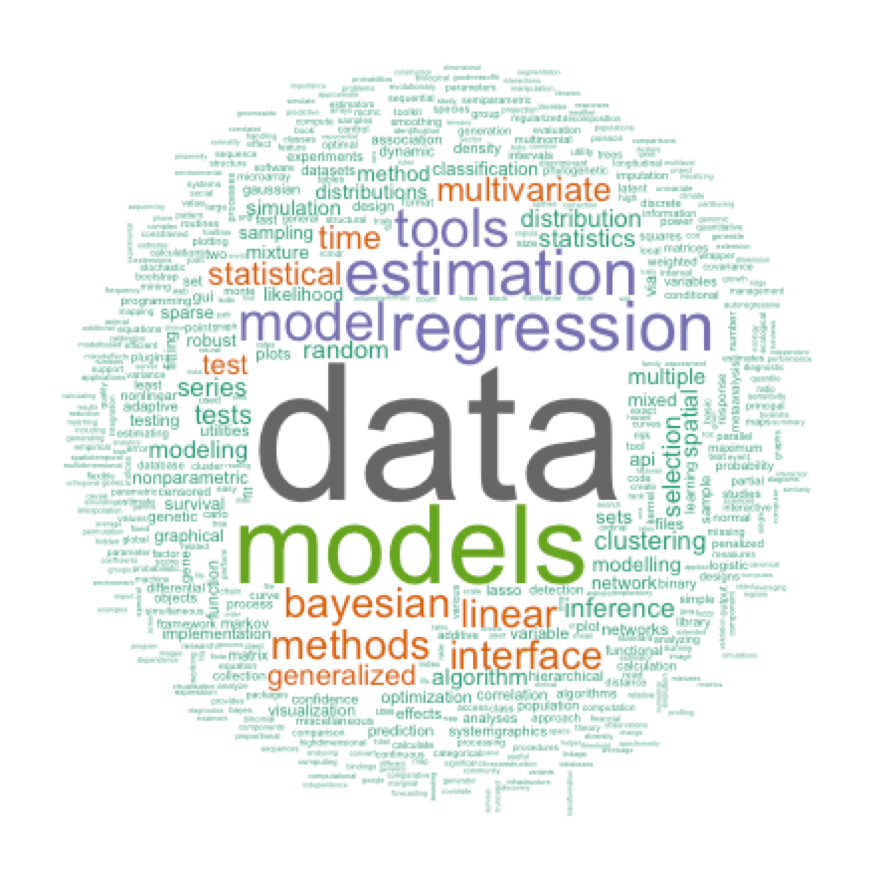
The Applied Statistics Research line at BCAM will contribute to create synergies between researchers from national and international institutions from different fields that require the use of statistical techniques for data modeling.
Our research is related to semi-parametric regression, multidimensional smoothing, (Bayesian) hierarchical models, random-effects models, longitudinal data, spatial and spatio-temporal modeling, functional data analysis, computational statistics, and data visualization tools and methods.
In particular, in the biomedical area, "Biostatistics" uses data to measure, understand and ultimately solve medical problems, by the use of statistical models and theory. Biostatistics is an exciting and versatile discipline contributing to all fields of medical research, evidence-based health care and decision-making. The increasing need of biostatistical support for the Basque Public Health Institutions, demands researchers in Biostatistics that not only support other researchers in biomedical and related sciences through statistical analyses and scientific support, but specially to contribute to high-impact research, excellence, innovation and training in statistical modeling.
The research line contributes with the Spanish National Network of Biostatistics (BIOSTATNET), a pioneer network led by applied statisticians from different institutions with own research projects and teaching experience in Biostatistics, working closely with biomedical researchers. We also actively collaborate with the Biostatistics group at University of the Basque Country (UPV/EHU) and other national and international institutions in order to address issues of mathematical and statistical theory and methodology to improve decision-making process. We aim to highlight and increase the role of Statistics and foster collaboration with our partners and promote professional development and training in the area of Applied Statistics.
The statistical modeling methodology developed by the group deals with those aspects of the analysis of data that are not highly specific to particular fields of study. Therefore, our research provides concepts and methods that will, with suitable modification, be applicable in many fields (e.g. Economics, Business, Engineering, Demography etc.) which demand a wide variety of data modeling and computational tools for the analysis of complex problems, particularly where a huge amount of data is collected.
Statistical Modelling for Recurrent Events in Sports Injury Research with Applications to Football InjuryData.
Spatio-temporal modelling of high-throughput phenotypic data
A general framework for prediction in generalized additive models
Hierarchical modelling of patient-reported outcomes data based on the beta-binomial distribution
npROCRegression: nukleoan oinarritutako ROC erregresio modelatze ez parametrikoa
Zenbait erregresio planteamendu ez parametriko aplikatzen ditu diagnostiko errendimenduaren (ROC) esparruan aldagaikideak txertatzeko.
Deskargatu hemen:
npROCRegression: Modelización de regresión ROC no paramétrica basada en kernel
Implementa varios enfoques de regresión no paramétrica para incluir información sobre covariables en el marco de características operativas del receptor (ROC).
Se puede descargar desde:
npROCRegression: Kernel-Based Nonparametric ROC Regression Modelling
Implements several nonparametric regression approaches for the inclusion of covariate information on the receiver operating characteristic (ROC) framework.
Download from:
PROreg: Patient Reported Outcomes Regression Analysis
Offers a variety of tools, such as specific plots and regression model approaches, for analyzing different patient reported questionnaires. Especially, mixed-effects models based on the beta-binomial distribution are implemented to deal with binomial data with over-dispersion (see Najera-Zuloaga J., Lee D.-J. and Arostegui I. (2017).
Download from:
PROreg: Análisis de regresión de los resultados comunicados por el paciente
Ofrece varias herramientas, como los gráficos específicos así como enfoques de modelos de regresión, para analizar diferentes cuestionarios comunicados por los pacientes. Se implementan especialmente los modelos de efecto mixto basados en la distribución beta-binomial para tratar datos binomiales con sobredispersión (véase Najera-Zuloaga J., Lee D.-J. y Arostegui I. (2017)).
Se puede descargar desde:
PROreg: pazienteek adierazitako emaitzen erregresioaren analisia
Hainbat tresna eskaintzen ditu, hala nola grafiko espezifiko eta erregresio ereduen planteamenduak, pazienteek erantzundako galdetegi desberdinak aztertzeko. Zehazki, banaketa beta-binomialean oinarritutako efektu mistoko ereduak aplikatzen dira gehiegizko dispertsioa duten datu binomialak lantzeko (ikus Najera-Zuloaga J., Lee D.-J. eta Arostegui I. (2017).
Deskargatu hemen:
SpATS: Spatial Analysis of Field Trials with Splines
Allows for the use of two-dimensional (2D) penalised splines (P-splines) in the context of agricultural field trials. Traditionally, the modelling of the spatial or environmental effect in the expression of phenotypes has been done assuming correlated random noise (Gilmour et al, 1997). We, however, propose to model the spatial variation explicitly using 2D P-splines (Rodriguez-Alvarez et al., 2016; arXiv:1607.08255). Besides the existence of fast and stable algorithms for estimation (Rodriguez-Alvarez et al., 2015; Lee et al., 2013), the direct and nice interpretation of the spatial trend that this approach provides makes it attractive for the analysis of field experiments.
Download from:
SpATS: Spatial Analysis of Field Trials with Splines
Permite la utilización de splines (p-splines) bidimensionales (2D) penalizados en el contexto de ensayos de campo agrícolas. Tradicionalmente, la modelización del efecto espacial o ambiental en la expresión de los fenotipos se ha realizado asumiendo un ruido aleatorio correlacionado (Gilmour et al, 1997). Sin embargo, nosotros proponemos modelizar la variación espacial explícitamente utilizando P-splines bidimensionales (Rodríguez-Alvarez et al., 2016; arXiv:1607.08255). Además de la existencia de algoritmos rápidos y estables para su estimación (Rodríguez-Alvarez et al., 2015; Lee et al., 2013), la interpretación directa y agradable de la tendencia espacial que proporciona este planteamiento hace que sea atractivo para el análisis de los experimentos de campo.
Se puede descargar desde:
SpATS: landa proben analisi espaziala spline-ekin
Bi dimentsioko (2D) spline penalizatuak (P-spline) erabiltzeko aukera ematen du nekazaritzako landa proben testuinguruan. Tradizionalki, fenotipoen adierazpenean efektu espazialak edo ingurumen efektuak modelatzeko, elkarri lotutako ausazko zaratak onartu izan dira (Gilmour et al, 1997). Hala ere, guk proposatzen duguna da aldakuntza espaziala modu esplizituan modelatzea 2D P-spline-ak baliatuta (Rodriguez-Alvarez et al., 2016; arXiv:1607.08255). Estimazioa egiteko algoritmo azkar eta egonkorrak izateaz gain (Rodriguez-Alvarez et al., 2015; Lee et al., 2013), planteamendu honek eskaintzen duen joera espazialaren zuzeneko interpretazio baliagarriari esker, landako probak aztertzeko planteamendu erakargarria dela esan daiteke.
Deskargatu hemen:
OpenTraffic
OpenTraffic is an open source platform for Traffic Incidences Data Analytics in Euskadi.
Authors: Gorka Kobeaga, Dae-Jin Lee
License: General Public License
HRQoL
HRQoL is an R package containing regression models with Beta-Binomial distribution for Health Related Quality of Life data
Authors: Josu Nájera, Dae-Jin Lee
License: General Public License
SAP
R package for fast estimation of multidimensional models with anisotropic penalties
Authors: María Xosé Rodriguez, Dae-Jin Lee, Thomas Kneib, María Durbán, Paul Eilers
License: General Public License
SOP
Pre-release version of a more general which includes SAP algorithm and implements adaptive smoothing in one a more dimensions
Authors: María Xosé Rodriguez, Manuel Oviedo, Dae-Jin Lee
License: General Public License
Placement
Personal computer
statgenHTP
High Throughput Phenotyping (HTP) Data Analysis
Authors: Emilie J Millet, Maria Xose Rodriguez Alvarez, Diana Marcela Perez Valencia, Isabelle Sanchez, Nadine Hilgert, Bart-Jan van Rossum, Fred van Eeuwijk, Martin Boer
License: Open source
spHDM
Supporting code for: "A two-stage approach for the spatio-temporal modelling of high throughput phenotyping data" (Scientific Reports)
Authors: Diana Marcela Pérez Valencia, María Xosé Rodríguez Álvarez, Martin Boer, Lukas Kronenberg, Andreas Hund, Llorence Cabrera Bosquet, Emillie Millet, Fred van Eeuwijk
License: Open source
spatio-temporal spHDM
Supporting code for: "A one-stage approach for the spatio-temporal modelling of high throughput phenotyping data" (BiorXiv, under review JABES)
Authors: Diana Marcela Pérez Valencia, María Xosé Rodríguez Álvarez, Martin Boer, Fred van Eeuwijk
License: Open source
TimeToEvent-InjurySim
The accompanying code repository for the scientific paper: "Zumeta-Olaskoaga, L., Weigert, M., Larruskain, J., Bikandi, E., Setuain, I., Lekue, J., … Lee, D.-J. (2021). Prediction of sports injuries in football: a recurrent time-to-event approach using regularized Cox models. AStA Advances in Statistical Analysis, 1–26. doi: 10.1007/s10182-021-00428-2"
Authors: Lore Zumeta-Olaskoaga (software developer), Maximilian Weigert (software developer)
Jon Larruskain, Eder Bikandi, Igor Setuain, Josean Lekue, Helmut Küchenhoff, Dae-Jin Lee (co-authors)
License: MIT
flex-mod-training-loads-recu-injuries
The accompanying code repository for the research paper: "Zumeta-Olaskoaga, L., Bender, A. and Lee, D.-J. Flexible modelling of time-varying exposures and recurrent events to analyze training loads effects in team sports injuries".
Authors: Lore Zumeta-Olaskoaga (software developer), Andreas Bender and Dae-Jin Lee (co-authors)
License: MIT
injurytools
Injury tools R package: "A Toolkit for Sports Injury Data Analysis"
Authors: Lore Zumeta-Olaskoaga (author, mantainer)
License: MIT