MCEN
Mathematical, Computational and Experimental Neuroscience
Explain how the brain processes and stores information, how these are disrupted under pathological conditions and develop novel technologies to treat neurodegenerative diseases.
The MCEN research group develops innovative research at the interface between Mathematical, Computational and Experimental Neuroscience. This involves developing novel theoretical and algorithmic tools, multiscale parsimonious models (biophysical and data-driven), neuroscientific experiments and data analytical tools to extract of invariant patterns from experimental and clinical observations.
The research goals are threefold: 1. To mathematically explain neurophysiological mechanisms that underlie the electro-chemical activity observed in experimental and clinical studies. This includes studying the neural code and explaining pathological electro-chemical brains states. 2. To develop novel drugs via state-of-the-art Artificial Intelligence methods in closed-loop with experiments (e.g. mini-brains) to treat neuro-degenerative diseases. 3. To develop novel tools based on mathematical control theory to track stability boundaries and unstable states directly from noisy data measured in closed-loop experiments. This will lead for example to alternative clinical therapies (via intelligent deep-brain stimulators) to treat epileptic patients that are not responsive to anti-epileptic drugs. Moreover, such technology will enable the development of machine-brain interfaces that are capable of efficiently communicating with the neural tissue. The hope is that some of these technologies will be commercially exploited by targeting specific industries.
Multiscale Mathematical Modelling:
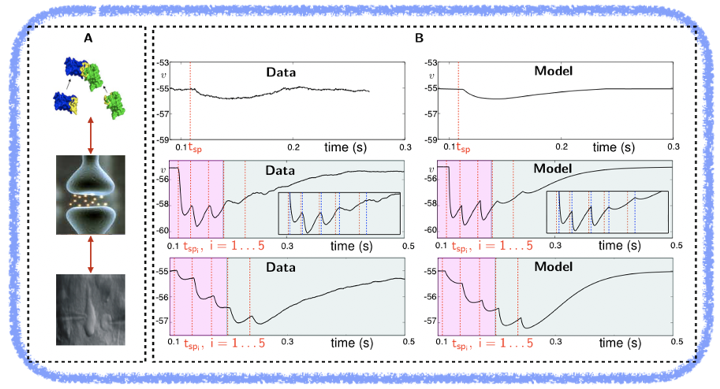
Example 1: Multiscale synaptic model that bridges the gap between protein-protein interaction (the SNARE-SM complex) and neuronal electrical activity measured by dual-whole cell recordings. The model explains how synaptic molecular machinery finely tunes the timing of neurotransmitter release via different modes (synchronous, delayed and spontaneous) and short-term plasticity (as experimentally characterized by 2013 Nobel Prize winner Prof. Thomas Südhof).
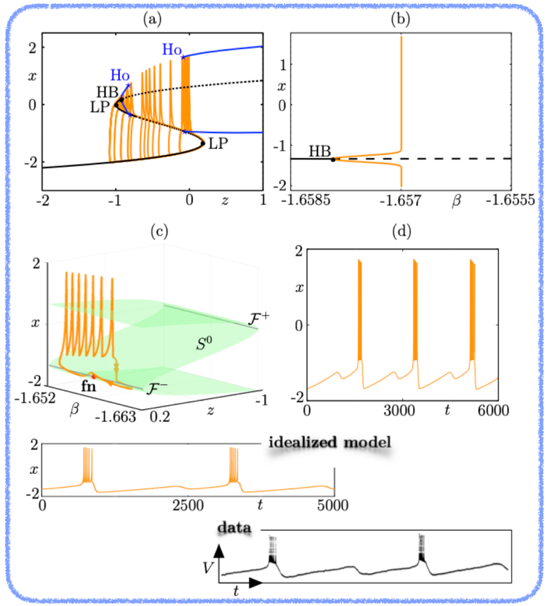
Example 2: A new theoretical framework for modelling complex multi-scale neuronal oscillations, with both non-trivial slow and fast components. This approach extends previous bursting oscillations classification schemes. This framework canonically explains complex electrophysiological data that escaped previous state-of-the-art bursting oscillations classification schemes. An example of such data is shown at the bottom panel (extracted from Roy et al., J. Neuro Physiol., 1984).
Drug Discovery for Neurodegenerative Diseases:
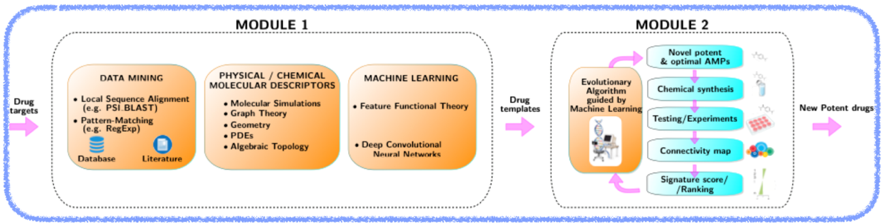
We are currently developing a new closed-loop computational-experimental pipeline for drug discovery for neurodegenerative diseases (in particular Alzheimer’s disease). This platform will involve the use of several state-of-the-art machine-learning techniques, which will result in an improved protocol to design new drugs.
Data-driven computational methods:
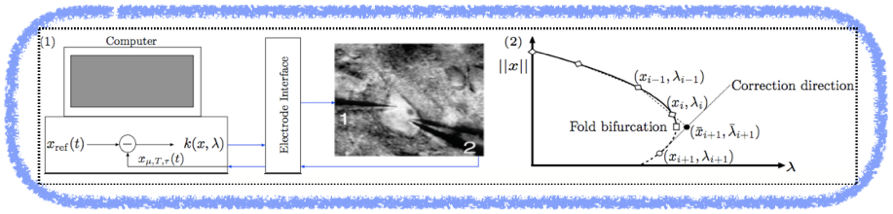
Example 1: We are developing a novel theoretical framework (CBCE method) that combines three well established theories, namely: Bifurcation Theory for dynamical systems, Pseudo-Arclength Numerical Continuation methods for tracking stability boundaries and Feedback Control Theory. These combined theories enable the implementation of a robust method that allows for direct tracking of nonlinear oscillations and stability boundaries directly from noisy experimental data (i.e. model-free) measured in closed-loop experiments such as dynamic-clamp. This has the potential to track stability boundaries between normal and pathological brain states (e.g. Epilepsy).
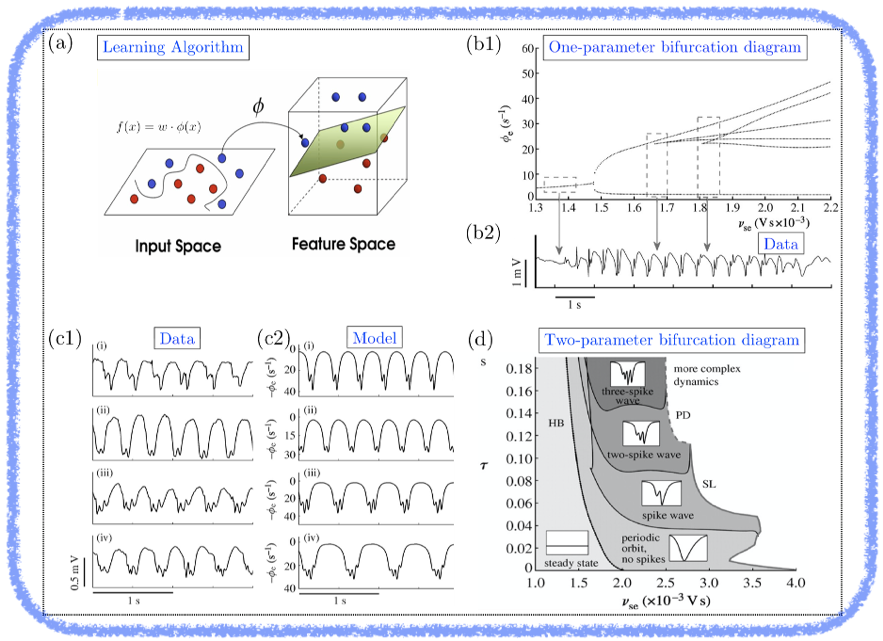
Example 2: Machine Learning (ML) has been successfully applied towards numerous applications, from engineering to biomedical data. Specifically, in ML with application to biomedical data, a typical problem is that of identifying classifiers amongst large data sets, for example, distinguishing from epileptic data, which patients are treatable by anti-epileptic drugs (AED) and those insensitive to AED. Techniques such as kernel methods and support vector machines lift (via an operator φ) the data to a higher dimensional space (i.e. feature space), where therein classification is made possible (see panel (a)). However, these techniques are unable to extract dynamical modes since they assume a static structure (i.e. atemporal) in the data (parameterised by fixed parameter ω). Therefore, typical ML methods have difficulty in dealing with data emerging from multi-scale spatio-temporal systems where complex oscillations vary across different epochs of data, e.g. observed in 3Hz EEG (poly)spike-wave data in absence seizure (see panel (b2)). To circumvent this we are interested in investigating the idea of endowing ML with knowledge about multi-scale models. To illustrate the point, we have developed a mean-field model that replicates 3Hz EEG (poly)spike-wave data (see panel (c1-c2)) and successfully relates changes in specific model parameters in a consistent manner to clinical recordings (see panel (b1-b2 and d)). Interestingly, fitting the model to epochs of data allows us to trace a pathway in a two-parameter bifurcation diagram (see panel (d)) that is consistent across different seizure episodes of the same patient. Moreover, the pathway (with some variability) dictates whether or not the patient is responsive to AED. This insight reveals that multi-scale spatio-temporal models with slowly-varying quantities (parameters) provide a robust technique for classifying dynamic varying complex data and is complementary to purely data-based approaches. The hope is that these techniques could be robust to be employed at a clinical setting.
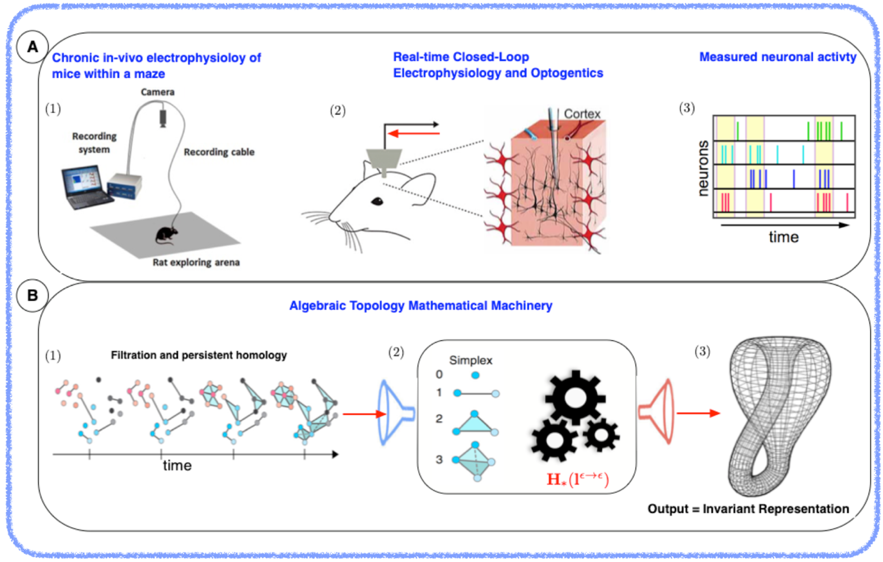
Example 3: We are currently data analytic methods to determine topological invariants from neuronal data, which could unravel the neural code. A: Mouse freely moving in a maze and chronically implanted e-cube system will measure the electrical activity of the entorhinal cortex. B: The Algebraic Topology machinery will algorithmically determine an invariant representation, that will allow us to decode the spatial navigation of the mouse. C: Simultaneously, in a closed-loop fashion we will control the relevant neurons via our CBCE method (mediated by optogenetics) to further understand the robustness of the invariant representation made by the mouse.
Code and data reproducing the paper "A unifying framework for mean field theories of asymmetric kinetic Ising systems"
Authors: Miguel Aguilera
License: GPLv3.0